A Guide to AI in Pharma: Part III
Our “Guide to AI in Pharma” blog series has covered the various artificial intelligence (AI) and machine learning (ML) techniques and introduced our framework for AI implementation. Next we’ll explore some of the factors that are making AI ready for primetime and how to get started.
Many executives are thinking about how best to integrate AI into their organizations to boost efficiency, and many more have already gotten started. A recent Gartner study revealed that 96% of tech leaders either had AI in their deployment pipelines or already initiated projects.
The healthcare sector is witnessing significant AI investment, projected to reach $45.2 billion by 2026 with a 44.9% compound annual growth rate. (CAGR) This growth is driven by AI's potential to reduce costs, improve patient care, and revolutionize drug development.
Healthcare and pharma leaders face several opportunities and challenges. Implementing a holistic AI solution involves numerous considerations, from building and training data models and cost to team needs and talent acquisition and much more.
In this final blog in our series, we share practical advice on getting your AI journey started.
Digital Transformation and AI: A New Era for Biopharma
The life sciences industry has experienced a decade's worth of digital transformation in just a few years, fundamentally changing how companies operate. As digital has become the standard way of doing business in biopharma, numerous new data sources have emerged. Digital technologies empower teams to deploy automation, intelligence, and data projects faster and more cost-effectively.
A perfect storm for AI adoption has formed, driven by the availability of large datasets, dramatic increases in computing power, and the storage, processing, and scalability offered by cloud computing. AI algorithms have been advancing rapidly, and a new generation of ML methodologies has increased the demand for reliable, scalable data. All these factors have accelerated AI technology development.
Before the pandemic pushed organizations toward digital-first strategies, digital was treated as a project, much like AI is today. However, this perspective has shifted dramatically over the past two years.
AI now provides unprecedented insights using large datasets across various parts of biopharma organizations. For instance, commercial teams can better understand their audiences and deliver more valuable experiences to meet their needs. This customer experience mindset has become commonplace.
Not only is AI development easier, with so many more use cases now in life sciences, but adoption has also been simplified. Just a few years ago, AI projects required locally installing and managing software. Now, Software-as-a-Service (SaaS) and Data-as-a-Service (DaaS) solutions use the cloud to seamlessly deliver data storage, integration, and processing. End-users can now access AI applications with only a network connection, rather than running applications locally on their hardware. This has allowed organizations to adopt and deploy AI more quickly, securely, and reliably.
"When adopting a SaaS offering providing ready-to-use AI, you need to consider the product's ability to scale and be adopted by your teams. But the most important aspect is trusting the offering, which is becoming easier to do as more platforms like ours are proven in the market"
-Mark Zou, Chief Product Officer, ODAIA
Key Considerations When Building and Scaling AI
Building and scaling AI solutions takes time, which is why you should start now. As you think about AI implementation, there are a number of technical and organizational elements that are important to get right. You're essentially building a new practice – from acquiring and structuring data to using predictive models to inform decision-making and measuring the impact of the practice on the business or organization.
Here are four top-line considerations when kickstarting your AI journey:
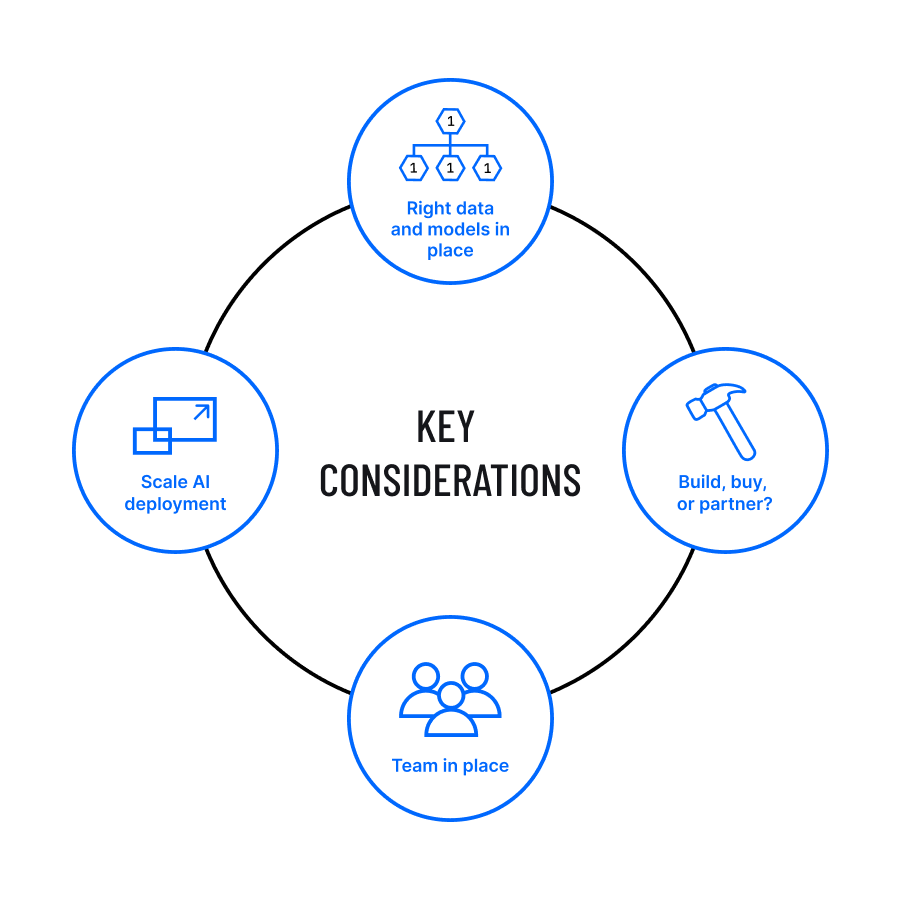
1. When implementing AI, do we build, buy, or partner?
The question of whether organizations should adopt AI solutions is no longer debatable. The focus has shifted to determining the best deployment methods and measuring AI's impact on operations and ROI.
When it comes to deployment, the most crucial decision after committing to AI is whether to build, buy, or partner. Therefore, it's imperative to decide whether your organization will purchase an off-the-shelf solution, develop its own, or collaborate with another organization to implement AI solutions.
This is a high-stakes decision. Many AI projects either never progress beyond the lab, are only partially implemented, or fail to gain end-user adoption.
Technology research firm Gartner, Inc. suggests that 85% of AI and machine learning (ML) projects fail to produce a business return.
Choosing the right implementation strategy for your organization can significantly increase the chances of your AI project succeeding. Looking forward, ODAIA is excited about a world where buy and build can coexist. Companies may choose to build some specific models which may then be leveraged by scalable off-the-shelf solutions.
2. Do we have the right data and models in place?
When considering AI deployment, it's crucial to understand the hierarchy of data science needs. Below is a roadmap for building a successful data science practice, from acquiring and structuring data to using predictive models to inform decision-making and measuring organizational impact:
- Data: High-quality data is the cornerstone of data science. This involves acquiring, cleansing, and structuring data for analysis. The best practice is to start this early. ODAIA has found that educating stakeholders about the data and its usage is critically important.
- Metrics: Define metrics that align with business goals to measure the success of your data science initiatives effectively.
- Analysis: Conduct exploratory analysis using statistical methods, data mining, and machine learning to uncover patterns and insights.
- Models: Build predictive models with techniques like regression, decision trees, and neural networks to guide decision-making.
- Decision-making: Use insights from models to inform decisions, communicate results clearly, and integrate them into business processes.
- Impact: Measure the effectiveness of your data science practice by tracking key performance indicators and monitoring the long-term success of your initiatives.some text
- The best practice is to define these indicators early and align on them as a team. You may have different levels of measurement – starting with end-user engagement, moving through behavior changes, and ending with ultimate business impact.
Having a plan in place is crucial to a successful deployment.
3. How do we scale AI deployment across the organization?
Scaling machine learning models from a single project to organization-wide implementation is a significant challenge. It involves processing large amounts of data, selecting the appropriate model architecture, using distributed training and deployment frameworks, and automating testing and monitoring processes.

The key to success requires you to not only create a machine learning model that can handle large datasets and provide accurate predictions, but one that uses real-world inputs to continuously improve. The ability to adapt ensures that predictions are not only accurate once, but repeatable over time.
Consider the following best practices:
- Data processing: Efficiently process large datasets using distributed frameworks like Apache Spark or Hadoop, which process data in parallel across multiple nodes.
- Feature engineering: Automate feature selection with techniques such as PCA (Principal Component Analysis), LDA (Linear Discriminant Analysis), or correlation-based methods to enhance model performance and manage large datasets effectively.
- Model selection: Choose the right model architecture. Regression modelling is ideal for numerical outcomes while classification models work better with categorical data. Ensuring that you have the resources and expertise to maintain the model post-selection.
- Model training: Leverage distributed training frameworks like TensorFlow or PyTorch to train models efficiently across multiple GPUs or nodes. Create a forward-looking plan that accounts for model re-training when new data is available.
- Model deployment: Use containerization tools such as Docker or Kubernetes to deploy models, ensuring they can handle high traffic across various environments. It is also worth considering the best way for your end-user to consume these outputs. Emailing out spreadsheets with hundreds of rows of predictions may reduce adoption of your AI.
- Testing and monitoring: Understand that models can become outdated quickly so using automated tools to test and monitor model performance to detect issues and send alerts in performance changes is crucial
When scaling AI solutions across an organization, it’s important to note that the ML model itself is a surprisingly small part of the overall solution. In a real world application, the ML model may only represent 5% or less of the overall code for the total ML production system. This is because ML production systems devote considerable resources to input data - collecting, verifying, and extracting features from data. Additionally, a serving infrastructure must be built in order to put the ML model’s predictions into practical use in the real world. Although these components lie outside of the ML model making the predictions, they are an essential part of a usable and scalable system.

By following these practices, you can effectively scale your machine learning code throughout your organization, ensuring robust performance and accurate predictions across large-scale implementations.
4. Do we have the team in place to ensure success?
Talent availability is a crucial consideration when embarking on an organization’s AI journey. A common misconception is that hiring a data scientist alone will suffice for building effective AI solutions. But, without the right support from those in other roles and with specialized expertise, data scientists may find that they spend more of their time on data management than developing machine learning models.
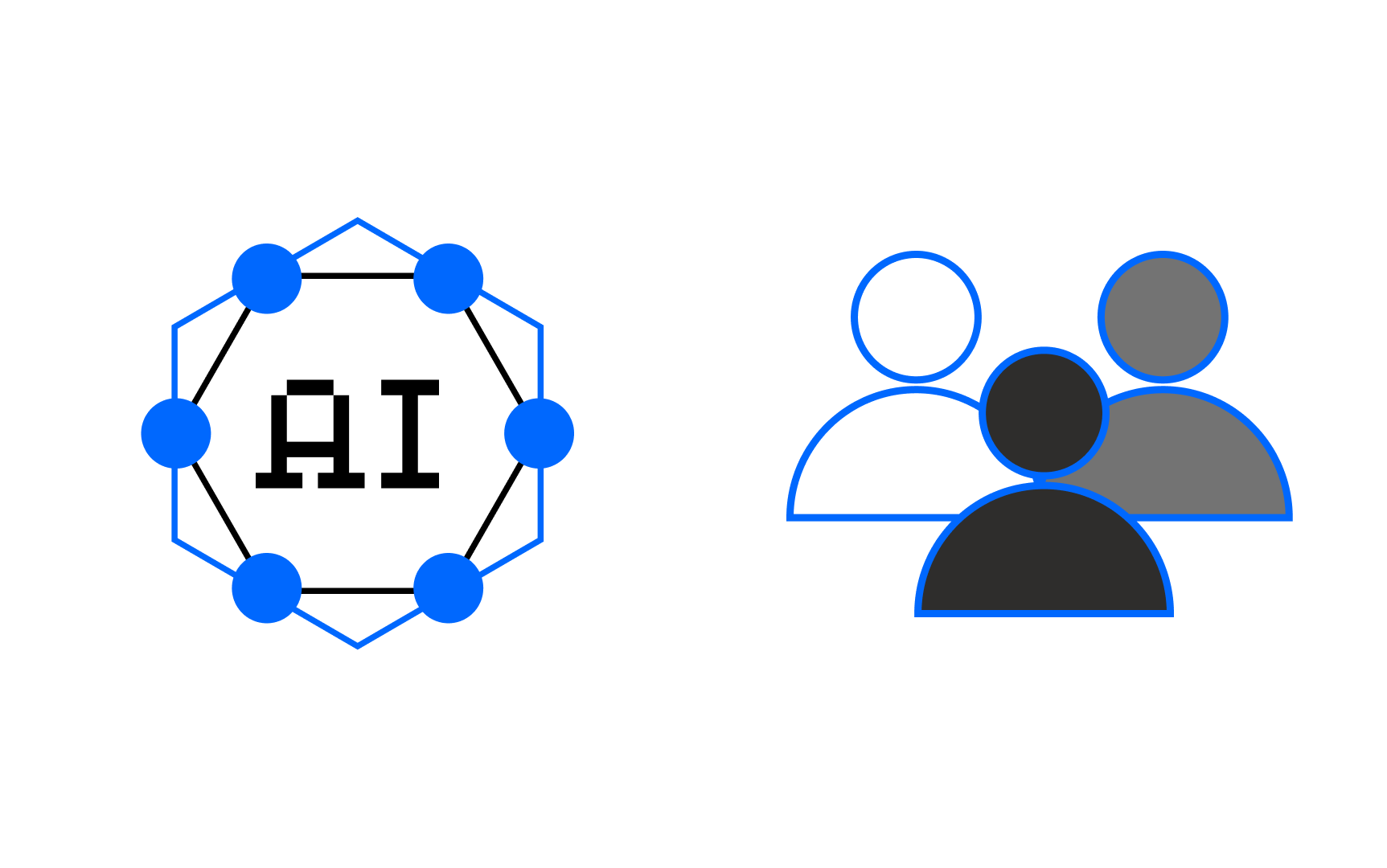
This is why beyond data scientists, the organization will need machine learning engineers, operations teams, database managers, solutions architects, UX/UI support and product managers. Each role contributes to the overall success of building, implementing, maintaining, and scaling AI solutions.
Key questions to consider when building your team include:
- Has our team tackled similar projects before?
- Who will champion the project within our team?
- Does our team possess greater depth of expertise, or can a partner or commercial vendor help?
- What is our technical hiring strategy?
- Who on our team has experience building an AI solution from scratch, from implementation to integration?
Many life science companies find it difficult to hire and retain talent while competing against tech giants. Leadership and team continuity needs to be factored in as well to make sure there is continuous and stable service. Retention strategies are just as important for both during and after an AI project.
Key Takeaways: Implementing AI in Pharma Organizations
Thanks for reading our “Guide to AI in Pharma” blog series. We hope you found it valuable as you consider when and how to implement AI in your organization.
Here are the key takeaways from the series:
- A holistic framework is essential to address multiple stakeholders’ needs
- AI tools are efficient and complement human work, augmenting rather than replacing it
- Adopting existing AI tools is now easier than ever, rather than building your own
- Addressing stakeholder and end-user needs is crucial for lasting organizational change
AI is continuously evolving, and to ensure you're meeting the needs of healthcare professionals and other constituents, it’s important to keep learning and adapting to the ever-changing technological landscape.