Innovation in artificial intelligence (AI) is advancing at a blistering pace. In the 18 months since OpenAI introduced ChatGPT, we've witnessed a proliferation of AI applications in our daily lives and its growing impact in the biopharma industry.
Today, nearly every technology vendor touts AI features and capabilities. However, many companies have contributed to the confusion surrounding AI through over-inflated claims about their expertise, or "AI washing.” This has only added to the noise around what AI truly is and how it can be effectively utilized.
So, it's understandable why many in the life sciences industry find AI perplexing. We’re here to help. Over the course of the next several weeks, we focus on a series of blogs as a “Guide to AI in Pharma.”
Let's dive into the world of AI by first defining it and then exploring some of the different AI techniques in use today.
Types of AI in analytics
AI is transforming the biopharma industry, offering significant advantages as organizations integrate it into their daily workflows. Analytics are entrenched across multiple areas of life sciences, from drug discovery and development to commercialization. Data analysts play a crucial role in interpreting historical data to inform decision-making in product development and commercial operations.
AI has expanded our capabilities beyond human limitations, enabling the analysis of vast amounts of data, faster learning, and more efficient problem-solving. AI can be trained on enormous datasets and applied to various aspects of analytics, including data processing, analysis, and decision-making.
Below are definitions of some increasingly common AI terms and techniques. These tools enable businesses to automate decision-making, identify patterns, and extract insights from large volumes of data, transforming how organizations operate and innovate.
AI systems are trained to perform tasks like perception, learning, motion, and language processing. These functions are achieved through coded algorithms often involving complex models. AI systems, particularly ML, are unique in their ability to learn patterns from data rather than following predefined rules.

What’s possible today with AI
Science fiction has long envisioned super-intelligent computers that rival or surpass human intelligence. This concept, known as General AI or Strong AI, describes systems that can not only interpret data but also extrapolate information and apply it across diverse fields, much like humans do. Some even speculate that machines one day could become self-aware and sentient.
However, current AI technology is far from this level of sophistication. We remain uncertain whether machines will ever truly reason, think, learn, perceive, move, and feel as humans do. General AI and Strong AI remain firmly in the realm of science fiction.
Today's discussions around AI typically refer to narrow AI—systems designed to address specific problems within defined domains that humans struggle to do on their own. Narrow AI is the most common application of AI technology today. Crucially, it relies on human training and input for its development and functionality.
Data science vs. machine learning
Data science is a multidisciplinary field that extracts insights from data using various techniques, including statistical analysis, data mining, and ML.
ML focuses on training algorithms to learn patterns in any data—from structured data such as numerical or categorical data to unstructured data like text, images, and audio—and make predictions based on its learning, rather than following explicit human instructions.
Just like there are multiple AI techniques, there are several types of ML that can be trained using a variety of algorithms, including decision trees, random forests, and neural networks. Here are the main types of ML approaches:
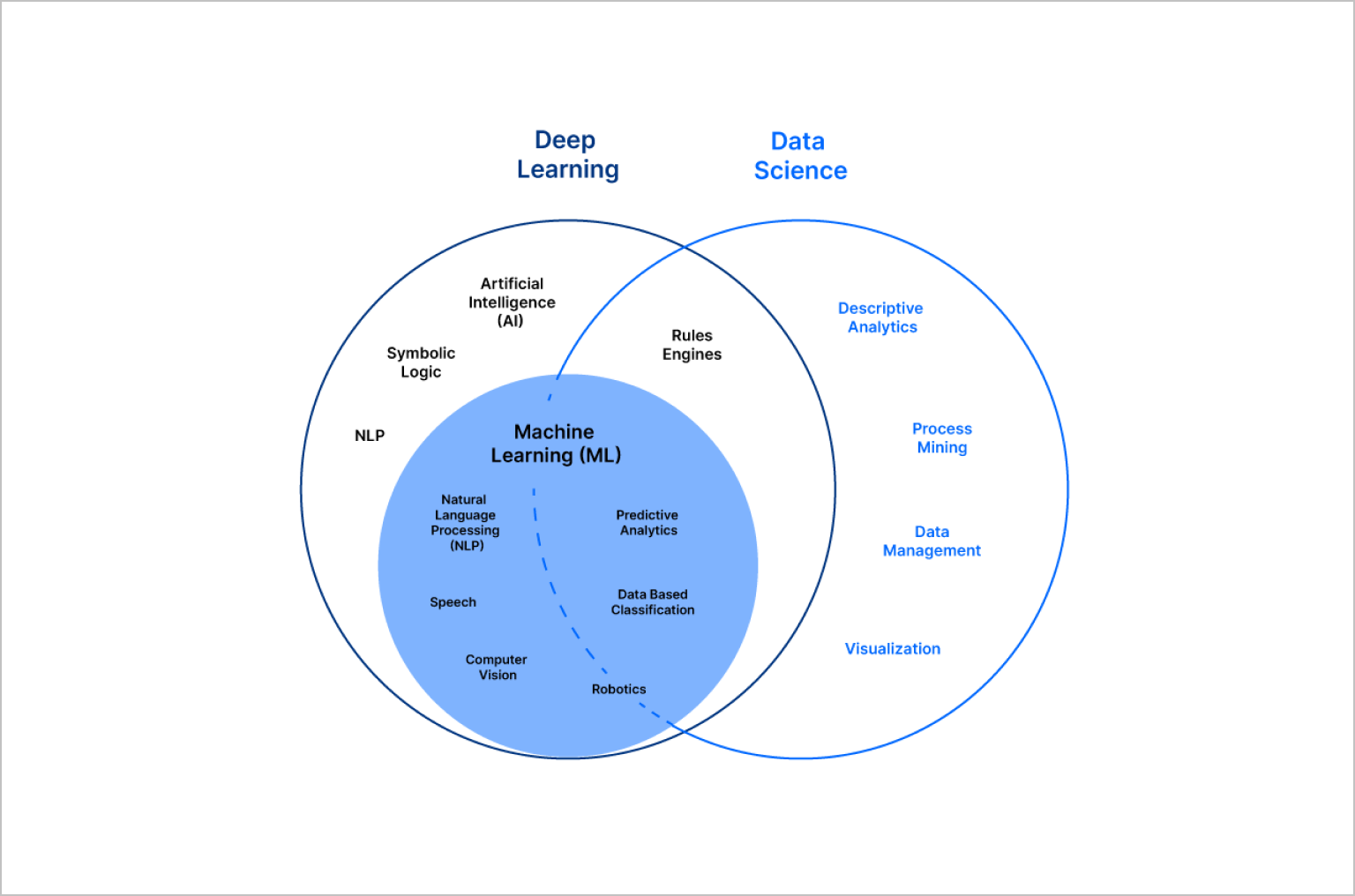
Data science and ML are interconnected, with ML serving as a key technique in data science. However, data science extends beyond ML, covering the entire data lifecycle from data collection and cleaning to analysis and visualization.
Rules-based analytics vs. AI
The main difference between rules-based analytics and true AI lies in their problem-solving approach.
Rules-based analytics is the traditional method of data analysis. It involves creating predefined rules or algorithms to analyze data and make decisions. Human experts with a deep understanding of the data identify patterns and formulate rules based on those patterns. Once rules are established, the system automates decision-making based on these predetermined instructions.
In contrast, true AI involves using algorithms and models to analyze data, identify patterns, and learn from data without explicit rules. AI systems can adapt to new data and changing situations by recognizing new patterns and modifying their outputs without human intervention, improving their decision-making capabilities over time. Rules-based systems, however, are constrained by their predetermined rules and require manual effort to change.
Data Analytics vs. Predictive and Prescriptive AI
Data analytics and predictive and prescriptive AI are both tools for extracting insights from data, but can differ in their focus and methodologies.
The key distinction between data analytics and predictive and prescriptive AI lies in the desired objective and methodology employed to achieve it. Data analytics aims to understand and interpret data while predictive and prescriptive AI are specialized techniques that fall under the umbrella of data analytics. AI requires specialized expertise in ML and data modelling and can be used to attain specific goals.
Endless possibilities with AI
The world of AI is nuanced and complex. Hopefully, after reading this, you have more confidence to speak to the different types of AI such as ML, and better understand how data science and analytics compare.
In our next blog, we discuss the importance of data, accuracy, and developing ethical AI solutions.
ODAIA helps commercial teams spend less time analyzing data, and more time engaging with the right customers.
